Enhancing Business Efficiency with Video Labeling Tool Machine Learning
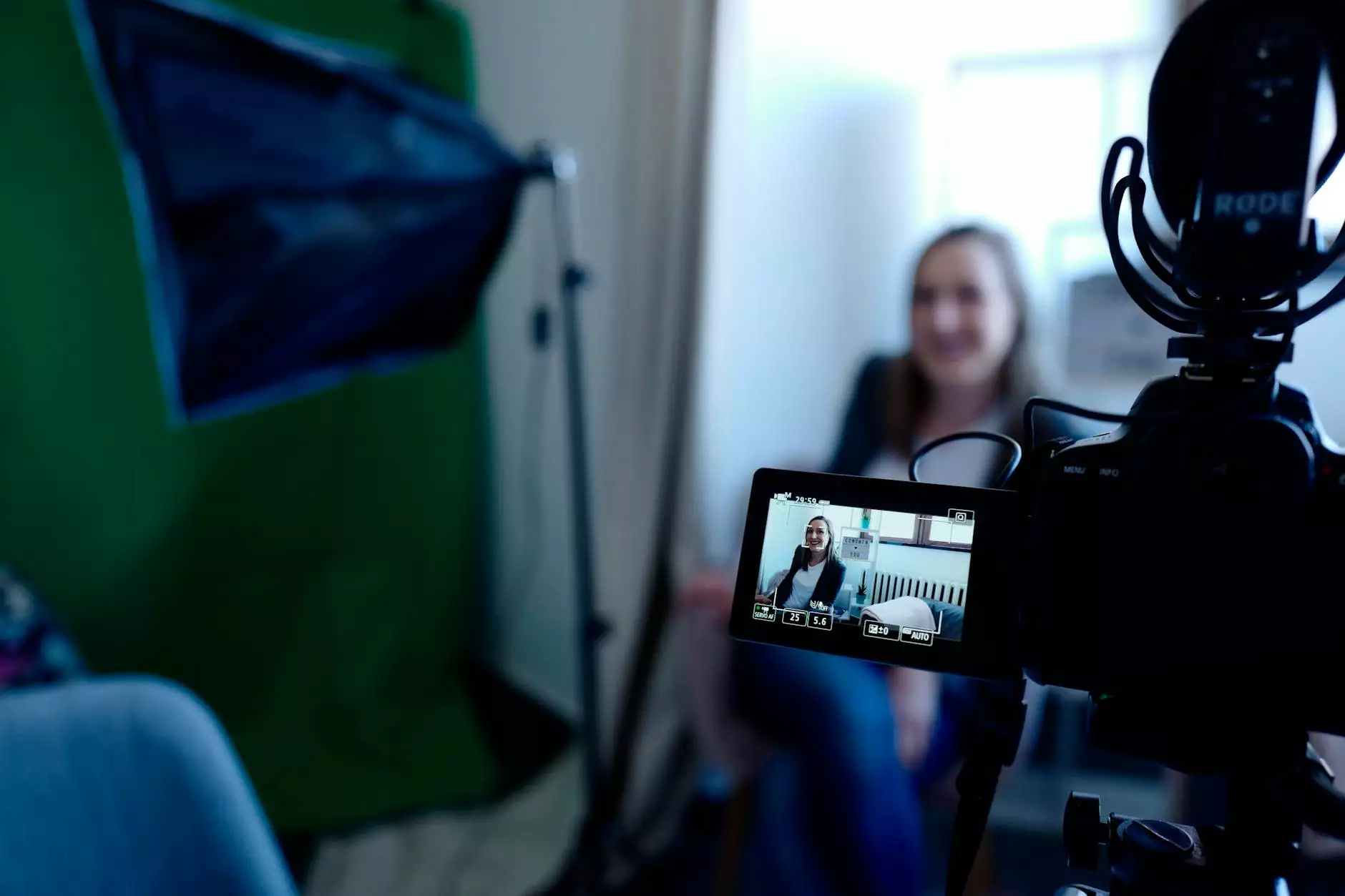
In an era where businesses rely heavily on data, the ability to efficiently handle and analyze that data is paramount. Video labeling tool machine learning has emerged as a vital solution to streamline data annotation, significantly impacting how companies operate and make decisions. In this article, we will delve deep into the functionalities, advantages, and the future of video labeling tools in the machine learning sphere.
Understanding Video Labeling Tool Machine Learning
Video labeling tool machine learning refers to the implementation of advanced technologies to process, annotate, and classify video data. This is especially crucial in fields such as artificial intelligence (AI), computer vision, and deep learning. The video labeling process involves the identification and tagging of objects, actions, and other elements within video content. This structured data is then utilized to train machine learning models, enabling them to learn and apply the knowledge in real-world applications.
The Importance of Data Annotation in Business
Data annotation serves as the foundation for machine learning models. Businesses across various sectors, from healthcare to automotive, utilize annotated data to refine their algorithms. Here are several reasons why data annotation is crucial:
- Quality Insights: Annotated data allows businesses to gather meaningful insights and make informed decisions.
- Improved Accuracy: Accurate labeling enhances the performance of machine learning models, leading to better predictions.
- Automation of Processes: By using annotated data, companies can automate processes that lead to increased efficiency.
- Competitive Edge: Businesses that leverage data annotation can gain a significant advantage over their competitors.
How Video Labeling Tool Machine Learning Works
Implementing a video labeling tool machine learning involves several key steps:
1. Data Collection
The first step involves gathering video data from various sources. This can include surveillance footage, user-generated content, or any other forms of video material relevant to the business application.
2. Preprocessing the Data
Before labeling, the video data must be prepared. This may involve editing techniques to enhance clarity, converting file formats, and segmenting videos into manageable clips.
3. Annotation Process
The core of a video labeling tool is the annotation phase, where objects, actions, or events are tagged within the video. Specific styles of annotations can include:
- Bounding Boxes: Enclosing objects in rectangular boxes to identify them easily.
- Semantic Segmentation: Classifying each pixel in the video based on its object class.
- Temporal Labeling: Marking events based on specific time frames within the video.
4. Quality Assurance
Post-annotation, it's critical to review and ensure that annotations are accurate. This may involve both automated checks and human reviews to minimize errors.
5. Feeding Data into Machine Learning Models
Once the video data is accurately labeled, it can be used to train machine learning models, which will learn to recognize patterns and make predictions based on the annotated information.
Benefits of Using Video Labeling Tools in Machine Learning
The integration of video labeling tools in machine learning brings numerous benefits to businesses:
- Increased Efficiency: Automated tools significantly reduce the time required for data labeling, enabling quicker project turnarounds.
- Scalability: These tools allow businesses to handle massive volumes of video data without compromising quality.
- Enhanced Collaboration: User-friendly interfaces in modern annotation tools facilitate teamwork among data scientists and annotators.
- Cost-Effectiveness: Implementing automated labeling tools can lead to significant cost savings in the long run.
Key Features of an Effective Video Labeling Tool
When selecting a video labeling tool machine learning, businesses should look for the following features:
- User-Friendly Interface: A straightforward and intuitive design allows users to focus on annotation without steep learning curves.
- Robust Annotation Capabilities: The tool should support various annotation styles suitable for diverse use cases.
- Integration with Machine Learning Frameworks: The tool should easily integrate with popular machine learning frameworks and libraries.
- Collaboration Features: The ability to share projects and collaborate in real-time fosters teamwork.
- Automation Options: Features like auto-labeling can greatly enhance efficiency and speed up the annotation process.
Choosing Keylabs.ai for Video Annotation
At Keylabs.ai, we understand the transformative power of video labeling tool machine learning in today’s business landscape. Our comprehensive data annotation platform offers:
1. Cutting-Edge Technology
We utilize state-of-the-art machine learning algorithms to enhance the accuracy and efficiency of video labeling.
2. Customized Solutions
Our platform is designed to cater to specific business needs, ensuring that our clients receive tailored services that address their unique challenges.
3. Expertise and Support
With a team of seasoned professionals, we provide continuous support throughout the annotation process, from data collection to model training.
4. Secure Environment
Security is a priority at Keylabs.ai. We ensure all data is handled with the utmost care, adhering to industry standards and practices.
Applications of Video Labeling Tool Machine Learning Across Industries
The impact of video labeling tools extends across various industries:
1. Healthcare
In the healthcare sector, video labeling is used for monitoring patient behavior through video feeds, aiding diagnosis, and enhancing telemedicine solutions. Accurate annotations help in training AI models to detect anomalies or specific health conditions.
2. Automotive
Self-driving cars utilize video labeling to identify road signs, pedestrians, and obstacles. The precision in labeling is crucial for the safety and functionality of autonomous vehicles.
3. Retail
In retail environments, video labeling can track customer behaviors, preferences, and interactions with products. The insights gained are invaluable for improving customer experience and optimizing marketing strategies.
4. Security and Surveillance
Video labeling assists in surveillance systems, enabling automatic detection of suspicious activities and streamlining monitoring processes for security personnel.
The Future of Video Labeling Tool Machine Learning
As we advance further into the digital age, the demand for effective video labeling tools is expected to grow exponentially. Future developments may include:
- Greater Automation: Anticipated advancements in AI and deep learning will lead to even more advanced automated labeling processes.
- Real-Time Annotation: The ability to annotate videos in real-time, enabling immediate analysis and decisions.
- Enhanced Integration: Better integration with IoT devices and smart systems to provide comprehensive data ecosystems.
- More Robust Security Features: Advancing security measures to handle sensitive data in various applications, particularly in healthcare and finance.
Conclusion
In conclusion, the video labeling tool machine learning is not just a trend; it represents a critical evolution in data processing and machine learning. As businesses increasingly rely on precise data annotation to guide their strategies and operations, investing in the right tools becomes essential. Keylabs.ai stands at the forefront of this technology, ready to assist businesses in harnessing the power of video labeling to enhance their capabilities, drive efficiency, and achieve success. Visit us at keylabs.ai and explore how we can redefine your data annotation processes.