Unlocking the Future of Machine Learning Data Labeling
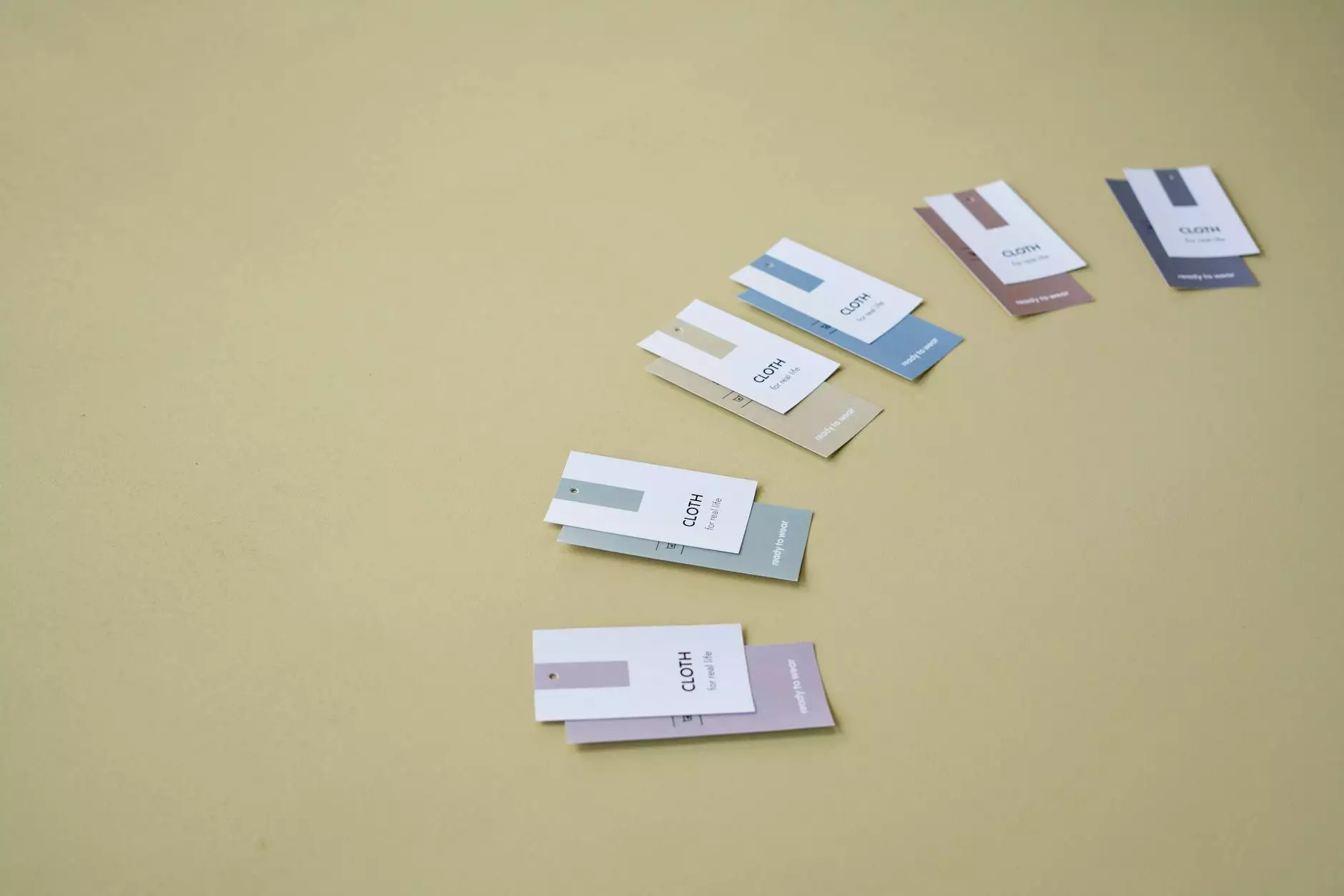
In the rapidly evolving landscape of artificial intelligence (AI) and machine learning, data labeling is a crucial component that cannot be overlooked. The quality of AI models is directly tied to the data they are trained on, making machine learning data labeling more important than ever. This article delves into the essentials of data annotation tools and platforms, how they enhance machine learning applications, and why partnering with experts like Keylabs.ai can elevate your business.
The Significance of Data Labeling in Machine Learning
Data labeling, also known as data annotation, refers to the process of adding informative tags to data, enabling machines to interpret and learn from it. This step is critical in creating datasets that machine learning algorithms can understand and utilize effectively. Without precise labeling, an AI’s ability to make accurate predictions diminishes significantly.
Types of Data Annotation
The data annotation process can be categorized into various types, each serving a specific purpose in the world of machine learning. Here are some common types of data annotation:
- Image Annotation: This involves identifying objects within images, such as drawing bounding boxes around cars, people, or animals. It is pivotal for computer vision applications.
- Text Annotation: This includes labeling parts of text, such as named entity recognition (NER), sentiment analysis, or categorization, which is particularly useful for natural language processing (NLP) tasks.
- Video Annotation: Here, labels are added to video segments to track movements or actions, making it essential for applications in surveillance, autonomous driving, and sports analytics.
- Audio Annotation: This encompasses the transcription and labeling of audio files, which aids in speech recognition systems and other audio-based AI applications.
Challenges in the Data Labeling Process
Despite its significance, machine learning data labeling comes with its own set of challenges. Some of the most prevalent challenges include:
- Quality and Consistency: Ensuring that data is labeled accurately and consistently across various annotators can be a daunting task.
- Scalability: As datasets grow exponentially, maintaining efficiency in data labeling becomes increasingly difficult, requiring advanced tools and strategies.
- Cost: Hiring skilled annotators or developing a robust in-house team can be expensive, particularly for startups and small businesses.
- Time-Consuming: Data labeling is often a lengthy process, which can slow down the overall AI development timeline.
Choosing the Right Data Annotation Tool
When looking for a data annotation tool, it's essential to consider the specific needs of your project. High-quality data annotation tools streamline the process, ensuring efficiency and accuracy. Here are some features to look out for:
User-Friendly Interface
A user-friendly interface minimizes the learning curve for annotators, allowing them to focus on data labeling rather than struggling with complex software.
Support for Various Data Types
Make sure the tool supports all necessary data types (images, videos, text, audio) to ensure versatility for future projects.
Collaboration Features
If your team consists of multiple annotators, look for tools that support collaboration. This helps maintain consistency and allows for a more integrated approach to data labeling.
Automated Features
Annotations can be enhanced with automated features to expedite the process. While human oversight is essential, machine learning algorithms can assist in suggesting labels based on already labeled data.
Quality Assurance Mechanisms
Select tools that provide built-in quality assurance features, ensuring a high degree of accuracy in the labeled data before it is fed into machine learning models.
Data Annotation Platforms: A Comprehensive Solution
Unlike standalone tools, data annotation platforms encompass broader solutions that include team management, multiple annotation types, and often, access to skilled annotators. Here are some benefits of utilizing a dedicated data annotation platform:
Access to Expert Annotators
Many data annotation platforms, such as Keylabs.ai, provide access to a pool of qualified annotators with domain expertise. This ensures that your data is not only accurately labeled but also contextualized by professionals who understand the intricacies of the data.
Integration with Machine Learning Frameworks
Effective data annotation platforms can seamlessly integrate with popular machine learning frameworks (like TensorFlow and PyTorch), enhancing the workflow and simplifying the model training process.
Customizable Workflows
Every project has unique requirements. A comprehensive annotation platform allows you to tailor the workflow according to your specific needs, whether that involves multiple data types or various levels of annotator expertise.
Scalability
As your project evolves, so too will your data annotation needs. A robust platform will accommodate the increasing volume of data without compromising on quality or speed.
Real-World Applications of Machine Learning Data Labeling
Understanding the real-world applications of machine learning data labeling can help businesses recognize its importance in their operations. Here are a few industries where data labeling is making significant impacts:
Healthcare
In the healthcare sector, machine learning models rely on annotated imaging data for tasks such as tumor detection and diagnosis. High-quality labeled datasets can improve patient outcomes by enabling more accurate diagnostic tools.
Autonomous Vehicles
Autonomous vehicles utilize data labeled in images and sensor inputs to understand their environment. This includes object detection, tracking, and recognizing road signs, all of which are vital for safe navigation.
Retail and E-commerce
Data labeling plays a crucial role in understanding customer behavior through sentiment analysis of consumer reviews and feedback. This information can further enhance product recommendations and marketing strategies.
Finance
In the financial sector, annotated datasets assist in developing algorithms for fraud detection, customer support automation, and risk assessment, ultimately maximizing efficiency and security.
Best Practices for Machine Learning Data Labeling
To ensure successful machine learning outcomes, following best practices for data labeling is essential. Here are some guidelines:
- Define Clear Guidelines: Ensure that annotators have a comprehensive understanding of the objectives and intended outcomes of the data labeling tasks.
- Implement Regular Training: Provide ongoing training for annotators to keep them updated on best practices and instructions as the project evolves.
- Utilize Feedback Loops: Incorporate feedback mechanisms to continuously improve both the annotators’ performance and the quality of the labeled data.
- Audit Data Regularly: Conduct periodic audits to ensure that the data labeling remains consistent and up to standard over time.
Conclusion
The importance of machine learning data labeling cannot be overstated. As businesses increasingly rely on AI to drive innovation and efficiency, the need for precise, high-quality data becomes paramount. By investing in robust data annotation tools and platforms, businesses can stay ahead of the curve while ensuring their AI models are trained on the best possible data. Leveraging expert capabilities, such as those provided by Keylabs.ai, can lead to superior outcomes, ensuring your journey into the world of AI is both effective and sustainable. Dive into the future of AI with confidence, armed with the right data and support!